AI in urology: The future is improved outcomes
AI is already solving complex problems in urologic oncology.
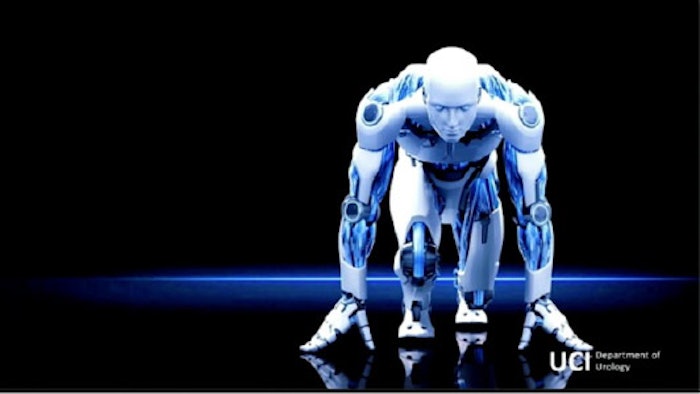
In layman’s terms, Saturday’s Panel Discussion on artificial intelligence (AI) was proof that if the future is not already here, it is not far away.
Moderated by Prokar Dasgupta, MD, professor and chair of Urology at King's College London, “Artificial Intelligence Application in Urology” featured presentations by Jaime Landman, MD, chair of the Department of Urology at the University of California, Irvine (UCI), and Andrew Hung, MD, an expert in robotic surgery for diseases of the adrenal gland, kidney, ureter, bladder and prostate at the University of Southern California (USC) in Los Angeles.
“While still in its infancy, AI has already upended entire industries and revolutionized the world as we know it,” Dr. Landman said in his presentation on applications in urologic imaging and outcomes. “It is essentially our clear duty to understand and apply this force to medicine and optimize patient outcomes for urologic disease.”
To function well, the algorithms that power AI need data. Fortunately, Landman said, because of the widespread use of electronic medical records, vast amounts of untapped data are ripe for harvest.
One area that is already using AI to solve complex problems is urologic oncology. Dr. Landman said that investigators at UCI are using AI to better manage prostate cancer by creating an architecture that will use the PI-RADS scoring system to accurately segment the prostate by zone.
“This is exactly the kind of problem for which AI is better equipped (to solve) than humans,” he said. “The AI can rapidly find higher-order patterns to predict outcomes while removing subjectivity from the process. Imagine simply taking an MRI, clicking a button and the computer spits out the likelihood of cancer quicker and more accurately than any human could. The foundation has been laid and we currently have the building blocks needed to do this. We just need to assemble them.”
In his presentation on assessing surgical competence with AI, Dr. Hung said his team at USC has used several years’ worth of surgical video and combined it with systems data from the da Vinci robot to develop a list of automated performance metrics (APMs). Dr. Hung and his colleagues then used the APMs to train a machine learning model to predict the length of stay after a robot-assisted radical prostatectomy (RARP).
“We were able to predict, with 85% accuracy, whether patients were going to spend one to two days in the hospital or more than two days after surgery was performed,” he said. “Over the years, we have increased our granularity and sophistication.”
In another study focused on predicting urinary continence recovery after RARP using a deep learning model, which was then used to evaluate surgeon's historical patient outcomes, Dr. Hung said the investigators concluded that using APMs and clinicopathological data, the deep learning models-based survival analysis (DeepSurv) model was able to predict continence after RARP. In this feasibility study, surgeons with more efficient APMs achieved higher continence rates at 3 and 6 months after RARP.
“Of the top 10 (negative factors affecting patient outcomes) that were most predictive, what we found was that all were surgeon factors, not patient factors,” he said.
Visit AUA2021 Daily News Online for more articles.